Probability-Flow ODE in Infinite-Dimensional Function Spaces
Kunwoo Na, Junghyun Lee, Se-Young Yun, Sungbin Lim
March, 2025
Abstract
Recent advances in infinite-dimensional diffusion models have demonstrated their effectiveness and scalability in function generation tasks where the underlying structure is inherently infinite-dimensional. To accelerate inference in such models, we derive, for the first time, an analog of the probability-flow ODE~(PF-ODE) in infinite-dimensional function spaces. Leveraging this newly formulated PF-ODE, we reduce the number of function evaluations while maintaining sample quality in function generation tasks, including applications to PDEs..
Publication
In The ICLR 2025 - Workshop on Deep Generative Model in Machine Learning - Theory, Principle and Efficacy (DeLTa)
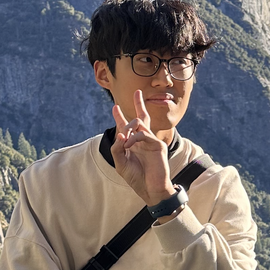
PhD Student
PhD student at GSAI, KAIST, jointly advised by Profs. Se-Young Yun and Chulhee Yun. Research focuses on interactive machine learning, particularly at the intersection of RLHF and preference learning, and statistical analyses of large networks, with an emphasis on community detection. Broadly interested in mathematical and theoretical AI and related problems in mathematics.