On the Estimation of Linear Softmax Parametrized Markov Chains
Kunwoo Na, Junghyun Lee, Se-Young Yun
June, 2024
Abstract
In reinforcement learning and deep learning, softmax parameterization is commonly used to represent discrete probability distributions.In this work, we study three possible softmax parametrizations of the transition matrix of the Markov chain. Through theoretical and empirical lenses, we provide several insights into the effect of such parametrizations on estimating the Markov transition matrix.
Publication
In Korea Computer Congress
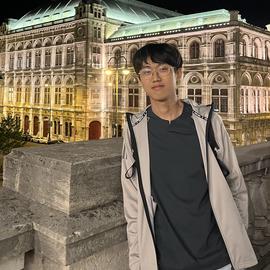
PhD Student
PhD student at GSAI, KAIST, jointly advised by Prof. Se-Young Yun and Prof. Chulhee Yun. Interested in mathematical and theoretical AI, i.e., essentially any machine learning challenges necessitating mathematical analysis. Recently focused on statistical problems arising from RLHF, including interactive machine learning and low-dimensional structure recovery.